China’s economy creates development opportunities, not ‘dumping shock,’ to world
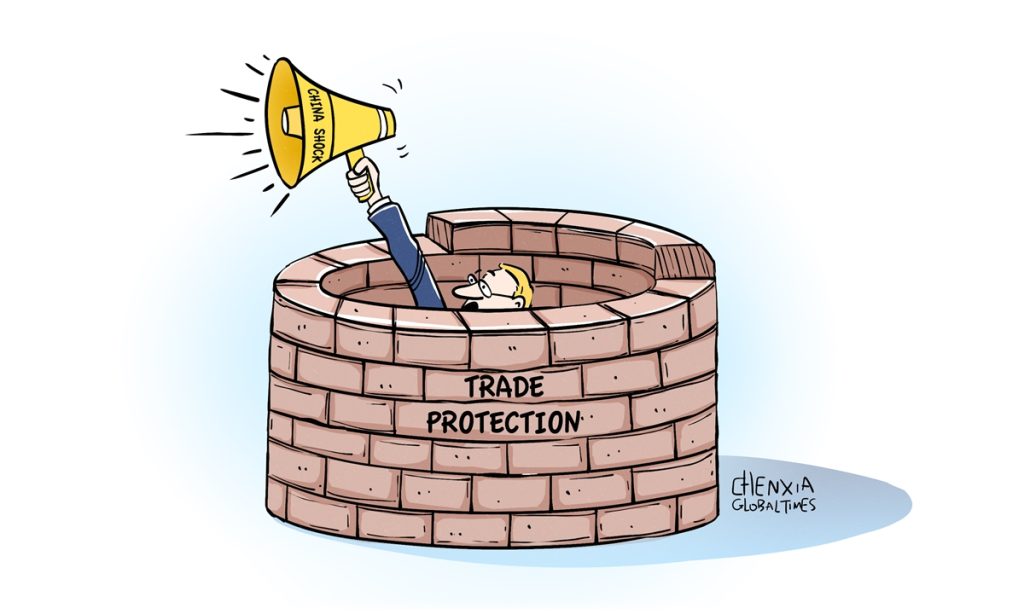
A root cause of why the US-launched smear campaign against the Chinese economy has become hysterical lies in the fact that only by vilifying China can the US-led West find an excuse for its unjust actions and attacks against the Chinese economy.
The Wall Street Journal (WSJ) published an article on Sunday with a sensational headline "The World Is in for Another China Shock." Its narrative sounded contagious and suited to the taste of political elites in Washington, especially when they resort to protectionist measures against ordinary Chinese goods.
Some Western media outlets are seeking to create a climate of public opinion in which the Western economy falls victim to China's "dumping" of cheap goods, so as to pave the way for further protectionist measures such as anti-dumping and anti-subsidy investigations into Chinese products.
With the WSJ serving as a vanguard, the US delivers a great deal of propaganda about "China flooding global markets with cheap goods," and uses that propaganda as a camouflage for its protectionist measures against China. It's an old trick by the US that should be condemned.
In recent years, Chinese industries have made steady progress in high-end manufacturing segments, including electric vehicles, batteries, solar panels, wind turbines and more. China's rise has increasingly raised concerns from some Western politicians, observers and media outlets. It's no surprise when they adopt protectionist trade measures to suppress Chinese enterprises, but they should not expect China to yield to these disgraceful acts.
It should be noted that China enters the global market with a peaceful and cooperative manner in line with global trade norms. China became a member of the World Trade Organization (WTO) in 2001. China is a staunch supporter of free trade.
The price advantage of Chinese goods can be attributed to multiple factors, including a complete industry chain, relatively low labor costs, and scientific and technological innovation. None of those seem to be factors related to China's "dumping of cheap goods."
The WSJ wrote in its article that the US and the global economy experienced a "China shock" - a boom in imports of cheap Chinese-made goods - in the late 1990s and early 2000s, and now, "a sequel might be in the making" as China doubles down on its exports. The author has a far more negative attitude toward the sequel's impact on the Western economy. This view is almost universal in the West at present.
Why is the West so much more afraid of the "sequel" that it needs to resort to protectionist measures to curb China's development? This reflects a lack of confidence by the West. In the late 1990s and early 2000s, the West experienced an upward growth cycle, taking an open mind toward globalization. However, after the 2008 financial crisis, and especially in more recent years, the Western economy has faced a series of challenges.
Amid the rise of anti-globalization sentiment and trade protectionism, some Western governments pin their hopes on putting up trade barriers to protect their own industries, although such measures will hinder industrial upgrading and the cultivation of emerging sectors.
It's a shame that some Western analysts claim "Chinese companies are flooding foreign markets with products they can't sell at home." The opposite appears to be true. Thanks to China's commitment to high-quality development and continuous progress in technological innovation, Chinese goods are increasingly competitive in the global market. Chinese ingenuity, diligence and adaptability have ensured sufficient supply of everything from daily necessities to high-quality tech products, allowing Chinese manufacturing to make great contribution to stabilizing global trade during a challenging time. China is by no means exporting excess capacity or dumping products they can't sell at home.
Meanwhile, the Chinese market is big enough to accommodate players from China, the West and other countries at the same time. Chinese officials have repeatedly stressed that foreign investment is welcome in China and the door to China will only open further.
China is the top trading partner for many countries in the world. The country is also a key market for many major international companies. There are many opportunities that foreign companies can take advantage of, including the large and fast-growing consumer market and investor-friendly policies. One thing is clear: China is important for multinational companies that want to be globally competitive.
Engaging with the Chinese market is seen as an opportunity rather than a risk. To the world economy, China is a contributor, rather than a saboteur that makes it suffer losses, or a "China shock."